Comparing artificial neural network algorithms for prediction of higher heating value for different types of biomass
Само за регистроване кориснике
2022
Чланак у часопису (Објављена верзија)
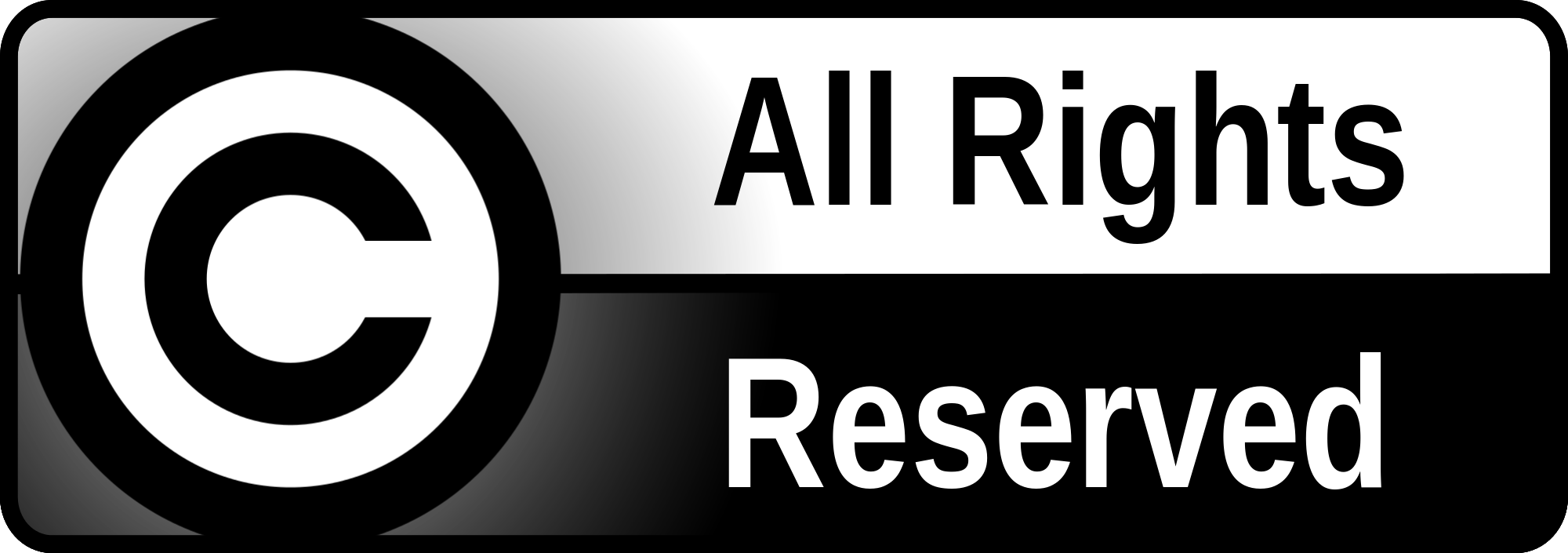
Метаподаци
Приказ свих података о документуАпстракт
A new set of software tools for the prediction of the higher heating values (HHV) of arbitrarily chosen biomass species is presented. A comparative qualitative and quantitative analysis of 12 algorithms for training artificial neural networks (ANN) which predict the HHV of biomass using the proximate analysis is given. Fixed carbon, volatile matter and ash percentage were utilized as inputs. Each ANN had the same structure but a different training algorithm (BFGS Quasi Newton, Bayesian Regularization, Conjugate Gradient—Powell/Beale Restarts, Fletcher–Powell Conjugate Gradient, Polak–Ribiére Conjugate Gradient, Gradient Descent, Gradient Descent Momentum, Variable Learning Rate Gradient Descent, Levenberg–Marquardt, One Step Secant, Resilient Backpropagation, Scaled Conjugate Gradient). To ensure an extended applicability of our results to a wide range of different biomass species, the data conditioning was based on diverse experimental data gathered from the literature, 447 samples ov...erall. Out of these, 301 datasets were used for the training, validation and testing by MathWorks MATLAB Neural Network Fitting Application and by custom designed codes, and 146 remaining datasets were used for the independent evaluation of all training algorithms. The HHV predictions of the ANN-based fitting functions were thoroughly tested and intercompared, to which purpose we developed a test suite which applies mean squared error, coefficient of the determination, mean Poisson deviance, mean Gamma deviance and Friedman test. The comparative analysis showed that several algorithms resulted in ANN-based fitting functions whose outputs correlated well with measured values of the HHV. All programming codes are freely downloadable.
Кључне речи:
Artificial neural networks / Biomass / Higher heating value / Proximate analysisИзвор:
Soft Computing, 2022Издавач:
- Springer Science and Business Media LLC
Финансирање / пројекти:
- Министарство науке, технолошког развоја и иновација Републике Србије, институционално финансирање - 200026 (Универзитет у Београду, Институт за хемију, технологију и металургију - ИХТМ) (RS-MESTD-inst-2020-200026)
DOI: 10.1007/s00500-022-07641-4
ISSN: 1432-7643; 1433-7479
Scopus: 2-s2.0-85142679102
Институција/група
IHTMTY - JOUR AU - Jakšić, Olga AU - Jakšić, Zoran AU - Guha, Koushik AU - Silva, Ana G. AU - Laskar, Naushad Manzoor PY - 2022 UR - https://cer.ihtm.bg.ac.rs/handle/123456789/5605 AB - A new set of software tools for the prediction of the higher heating values (HHV) of arbitrarily chosen biomass species is presented. A comparative qualitative and quantitative analysis of 12 algorithms for training artificial neural networks (ANN) which predict the HHV of biomass using the proximate analysis is given. Fixed carbon, volatile matter and ash percentage were utilized as inputs. Each ANN had the same structure but a different training algorithm (BFGS Quasi Newton, Bayesian Regularization, Conjugate Gradient—Powell/Beale Restarts, Fletcher–Powell Conjugate Gradient, Polak–Ribiére Conjugate Gradient, Gradient Descent, Gradient Descent Momentum, Variable Learning Rate Gradient Descent, Levenberg–Marquardt, One Step Secant, Resilient Backpropagation, Scaled Conjugate Gradient). To ensure an extended applicability of our results to a wide range of different biomass species, the data conditioning was based on diverse experimental data gathered from the literature, 447 samples overall. Out of these, 301 datasets were used for the training, validation and testing by MathWorks MATLAB Neural Network Fitting Application and by custom designed codes, and 146 remaining datasets were used for the independent evaluation of all training algorithms. The HHV predictions of the ANN-based fitting functions were thoroughly tested and intercompared, to which purpose we developed a test suite which applies mean squared error, coefficient of the determination, mean Poisson deviance, mean Gamma deviance and Friedman test. The comparative analysis showed that several algorithms resulted in ANN-based fitting functions whose outputs correlated well with measured values of the HHV. All programming codes are freely downloadable. PB - Springer Science and Business Media LLC T2 - Soft Computing T1 - Comparing artificial neural network algorithms for prediction of higher heating value for different types of biomass DO - 10.1007/s00500-022-07641-4 ER -
@article{ author = "Jakšić, Olga and Jakšić, Zoran and Guha, Koushik and Silva, Ana G. and Laskar, Naushad Manzoor", year = "2022", abstract = "A new set of software tools for the prediction of the higher heating values (HHV) of arbitrarily chosen biomass species is presented. A comparative qualitative and quantitative analysis of 12 algorithms for training artificial neural networks (ANN) which predict the HHV of biomass using the proximate analysis is given. Fixed carbon, volatile matter and ash percentage were utilized as inputs. Each ANN had the same structure but a different training algorithm (BFGS Quasi Newton, Bayesian Regularization, Conjugate Gradient—Powell/Beale Restarts, Fletcher–Powell Conjugate Gradient, Polak–Ribiére Conjugate Gradient, Gradient Descent, Gradient Descent Momentum, Variable Learning Rate Gradient Descent, Levenberg–Marquardt, One Step Secant, Resilient Backpropagation, Scaled Conjugate Gradient). To ensure an extended applicability of our results to a wide range of different biomass species, the data conditioning was based on diverse experimental data gathered from the literature, 447 samples overall. Out of these, 301 datasets were used for the training, validation and testing by MathWorks MATLAB Neural Network Fitting Application and by custom designed codes, and 146 remaining datasets were used for the independent evaluation of all training algorithms. The HHV predictions of the ANN-based fitting functions were thoroughly tested and intercompared, to which purpose we developed a test suite which applies mean squared error, coefficient of the determination, mean Poisson deviance, mean Gamma deviance and Friedman test. The comparative analysis showed that several algorithms resulted in ANN-based fitting functions whose outputs correlated well with measured values of the HHV. All programming codes are freely downloadable.", publisher = "Springer Science and Business Media LLC", journal = "Soft Computing", title = "Comparing artificial neural network algorithms for prediction of higher heating value for different types of biomass", doi = "10.1007/s00500-022-07641-4" }
Jakšić, O., Jakšić, Z., Guha, K., Silva, A. G.,& Laskar, N. M.. (2022). Comparing artificial neural network algorithms for prediction of higher heating value for different types of biomass. in Soft Computing Springer Science and Business Media LLC.. https://doi.org/10.1007/s00500-022-07641-4
Jakšić O, Jakšić Z, Guha K, Silva AG, Laskar NM. Comparing artificial neural network algorithms for prediction of higher heating value for different types of biomass. in Soft Computing. 2022;. doi:10.1007/s00500-022-07641-4 .
Jakšić, Olga, Jakšić, Zoran, Guha, Koushik, Silva, Ana G., Laskar, Naushad Manzoor, "Comparing artificial neural network algorithms for prediction of higher heating value for different types of biomass" in Soft Computing (2022), https://doi.org/10.1007/s00500-022-07641-4 . .